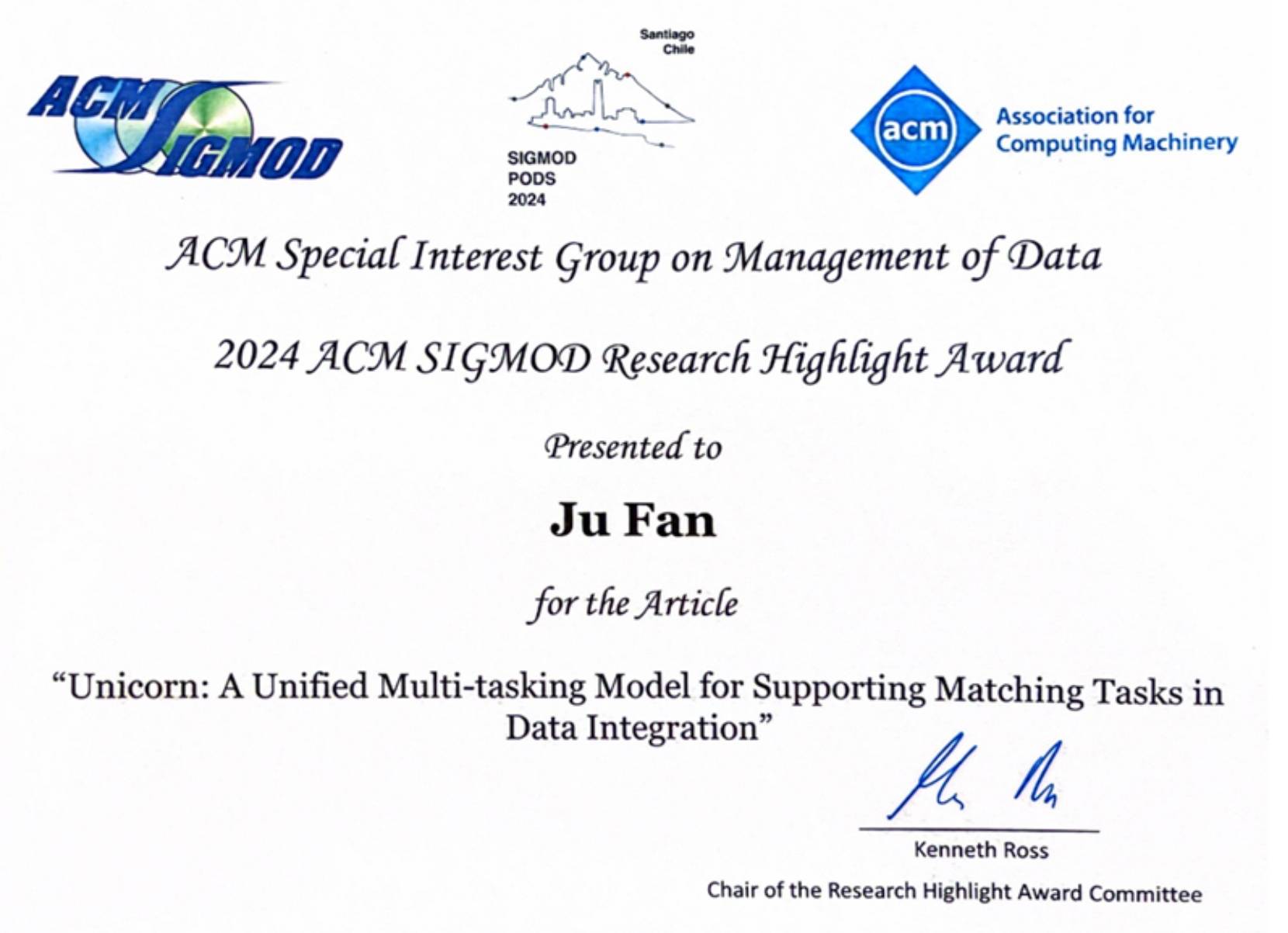
renmin university of china has won the 2024 acm sigmod research highlight award. this award is presented by the special interest group on management of data (sigmod) of the association for computing machinery (acm) to recognize projects that exemplify core database research.
the first author of the award-winning paper, titled “unicorn: a unified multi-tasking model for supporting matching tasks in data integration,” is jianhong tu, a master student of the class of 2020 from the school of information at renmin university of china. her advisors are prof. ju fan and prof. xiaoyong du from the same institution. this marks the first time renmin university of china has won this award as the primary institution. the paper, which was originally published at sigmod 2023, isalso a collaborative effort with the beijing big data center, and the tools developed based on this research have been successfully utilized in real-world applications in beijing.
the sigmod research highlight award, which was established in 2016, aims to showcase a set of research projects that exemplify core database research. in particular, these projects address an important problem, represent a definitive milestone in solving the problem, and have the potential of significant impact. the initiative of the sigmod research highlights also aims to make the selected works widely known in the database community, to the industry partners, and potentially to the broader acm community. the selection process for the award is highly selective. the award selection committee requested nominations from all top database conferences held in 2023 as well as from the sigmod community. then the award selection committee discussed all nominated papers and finally selected a subset of the papers that best met the selection criteria.only about ten papers receive this award annually. renmin university of china is the third mainland university to win this award, following tsinghua university and shanghai jiao tong university.
paper overview:
data integration, a fundamental problem in data management, plays a crucial role in many applications, such as big data analytics, knowledge graph construction, and data preparation for ai systems. data matching, is a most challenging problem in data integration, aiming to determine the semantic equivalence of heterogeneous data from multiple sources. over the past four decades, various fields such as databases, ai, semantic web, and data mining have studied data matching from different perspectives, introducing a varity of tasks like schema matching, entity matching, ontology alignment, column type annotation, etc. however, current researches mainly focus on designing specialized models for single matching task or dataset, lacking a general solution for different types of matching tasks. this paper introduces unicorn, a unified model supporting multiple matching tasks, with the advantage of integrating diverse matching tasks into an end-to-end model. its multi-task learning strategy enables knowledge sharing and mutual enhancement among tasks. experiments across seven common data matching tasks show that unicorn outperforms task-specific and dataset-specific models in terms of both matching accuracy and generalization capability.
introduction to the research group:
professor ju fan's research group, affiliated with the school of information and the key laboratory of data engineering and knowledge engineering of the ministry of education at renmin university of china, has conducted extensive research on data management under the guidance of professor xiaoyong du. the research interests of the research group are in general area of data management, and the current research focuses on building next-generation data preparation systems.in recent years, the team has published more than 60 papers at top conferences/journals, including sigmod, vldb, icde and vldb journal, and has actively colloaborated with industrial partners, such as beijing big data center, huawei, alibaba and tencent.